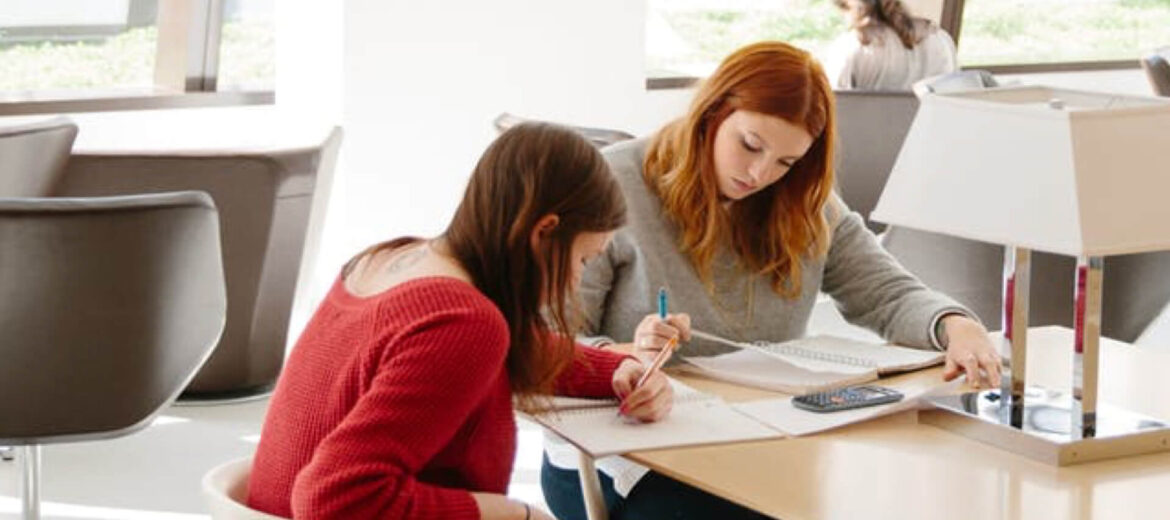
About The Event
A 6-hour workshop taught by Steve Porter, Ph.D.
Causal inference workshop series discount!
Register for this along with our workshop on Regression discontinuity designs for evaluating programs and policies for only $350 (would normally cost $450 for both). If you are interested in the workshop series, email us at mail@percontor.org for discount codes.
You may also be interested in our introduction to binary logistic regression class.
Overview:
Propensity score analysis (also known as “matching”) is a popular way to estimate the effects of programs and policies on outcomes. Yet researchers face a dizzying array of choices, in terms of particular matching techniques to use, as well as many different options for implementing a specific technique.
This workshop provides a concise introduction to matching for the applied researcher. Rather than cover every possible matching technique, we will focus on nearest neighbor matching (one of the most popular approaches) and inverse propensity weighting, a simple and powerful matching approach that can be used without any specialized software.
Expected outcomes:
By the end of the workshop, participants should understand why matching is preferred over regression, the major concepts underlying the counterfactual theory of causality, the major issues with implementing nearest neighbor matching, and whether they should estimate the average treatment effect or treatment effect for the treated for their particular research application. Most importantly, they should be able to immediately begin using inverse propensity weighting in their research, using any statistical software program.
Pricing and schedule:
Series discount – Register for this along with our workshop on Regression discontinuity designs for evaluating programs and policies for only $350 (would normally cost $450 for both). If you are interested in the workshop series, email us at mail@percontor.org for discount codes.
We offer $50 discounts for graduate students and $25 discounts for multiple workshop enrollments.
The main workshop material is scheduled for six hours. Time permitting, Dr. Porter will answer questions about participants’ specific research projects. Participants can ask questions via chat, microphone, or telephone. In order to allow sufficient time for questions, the number of workshop participants is limited to 30.
In order to use psmatch2, part of the presentation will use Stata. Stata is not required to participate, and the technique of inverse propensity weighting can be used in any software package that uses survey weights (e.g., SAS, SPSS and R).
Who should attend?
The target audience is researchers who typically use multiple regression, logistic regression and hierarchical linear modeling in their research and 1) wish to know why matching has become popular, and 2) how to use matching in their research. Participants should have a good understanding of multiple regression. Familiarity with logistic regression is helpful but not required. If you want to learn about logistic regression, consider our class on binary logistic regression.
Agenda:
- Advantages of matching over regression and other linear models
- Rubin’s Causal Model – the counterfactual approach to causality
- Understanding treatment effects for research projects
- Implementing nearest neighbor matching by hand and with psmatch2
- Choosing the right variables for the propensity model
- Advantages of inverse propensity weighting over all other matching techniques
- Implementing inverse propensity weighting using survey weight commands
- Common mistakes to avoid when matching